
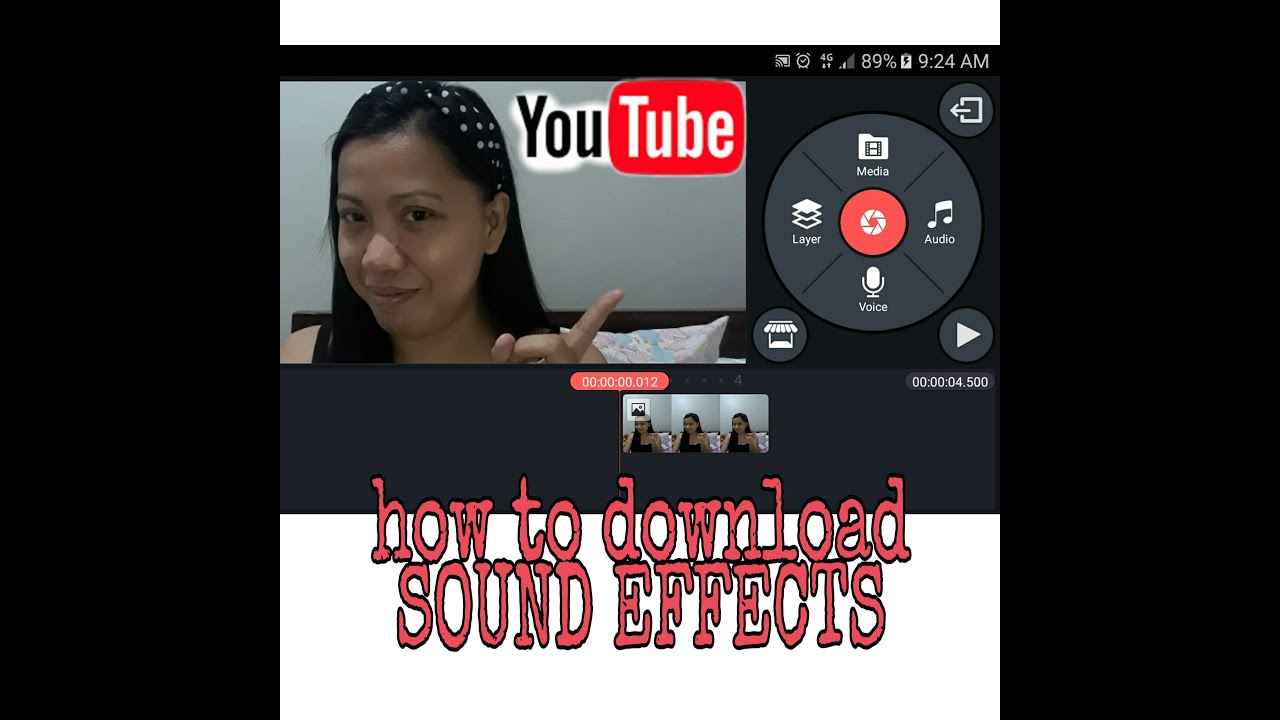
The application of ML – in this case, a Deep Neural Network (DNN) model – to the captioning task presented unique challenges.

In this post, we discuss the backend system developed for this effort, a collaboration among the Accessibility, Sound Understanding and YouTube teams that used machine learning (ML) to enable the first ever automatic sound effect captioning system for YouTube.Ĭlick the CC button to see the sound effect captioning system in action.
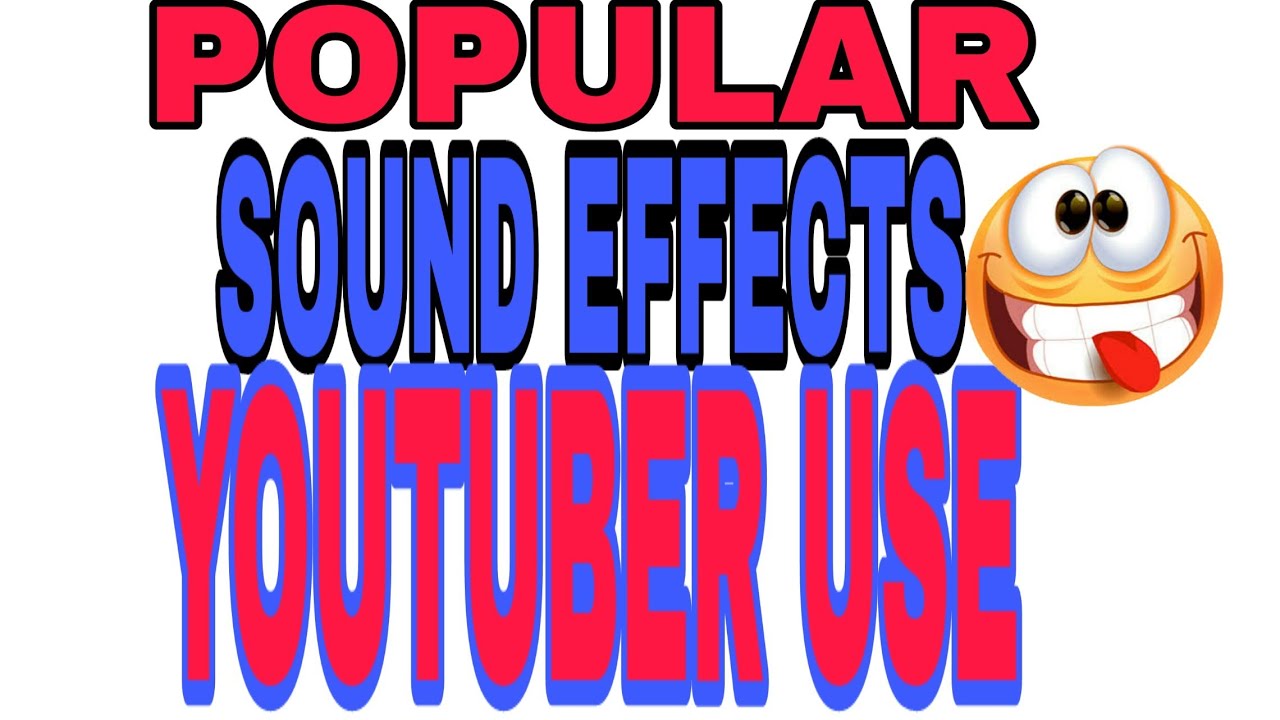
To address this, we announced the addition of sound effect information to the automatic caption track in YouTube videos, enabling greater access to the richness of all the audio content. However, without similar descriptions of the ambient sounds in videos, much of the information and impact of a video is not captured by speech transcription alone. Since 2009, YouTube has provided automatic caption tracks for videos, focusing heavily on speech transcription in order to make the content hosted more accessible. These ambient sounds create context that we instinctively respond to, like getting startled by sudden commotion, the use of music as a narrative element, or how laughter is used as an audience cue in sitcoms. Its importance as a communication medium via speech is obviously the most familiar, but there is also significant information conveyed by ambient sounds. The effect of audio on our perception of the world can hardly be overstated. Posted by Sourish Chaudhuri, Software Engineer, Sound Understanding
